AI in Healthcare: Promise and Pitfalls
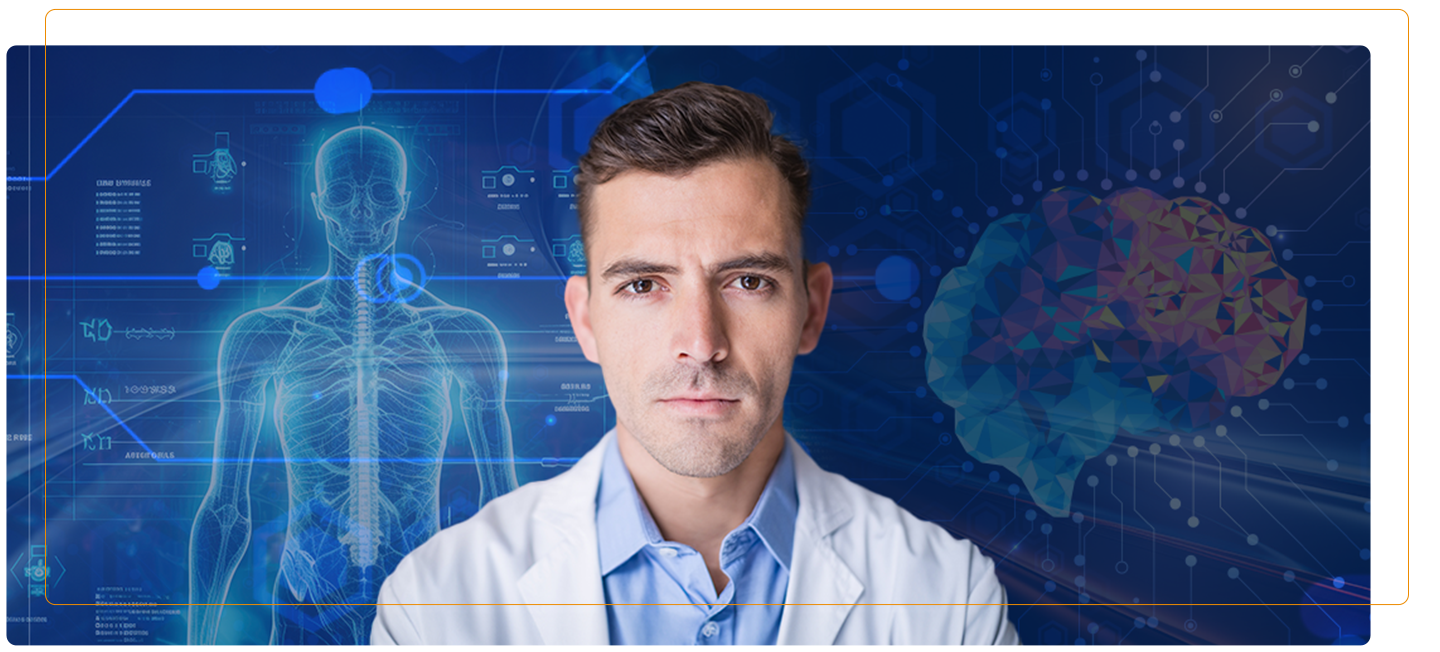
Executive Summary
Healthcare is a powerful example of how artificial intelligence (AI) is transforming the world. There is real promise in AI-driven care, ranging from optimizing scheduling and streamlining operational processes to facilitating diagnoses, tailoring treatment plans, and personalizing care. That promise, however, is matched by confusion among patients, consumers, and healthcare professionals about what AI is, the benefits and risks it carries, and the ethical concerns it will continue to raise as usage grows. Our research indicates that building trust requires health systems to clearly explain what AI is – and their vision for using it – to patients and communities as well as employees and affiliated staff. This nSight highlights five key points:
- Nearly 75% of healthcare consumers do not have a clear sense of how AI is used in healthcare.
- There are myriad uses of AI in healthcare and it’s important to think carefully and explicitly about how each one impacts both patients and care teams.
- While people are appropriately cautious about AI, those who report familiarity with AI are more likely to report being hopeful, excited, and confident about its use.
- Trust in the use of AI for various clinical functions is markedly higher for consumers who report familiarity with AI – but even they trust doctors alone more than doctors using AI.
- Trust in care providers and trust in AI will be increasingly intertwined, and education will need to address its promise, as well as potential pitfalls and how those pitfalls will be handled.
What is AI?
AI means many things to many people. Ask 10 AI experts to define AI, and you’re likely to get unique, albeit overlapping answers. Ask 10 non-experts about AI, and you might get a bunch of blank stares. Ask ChatGPT about AI in healthcare, and you get this:
Artificial intelligence (AI) in healthcare refers to the use of advanced technology, particularly machine learning algorithms and other computational techniques, to analyze complex medical data, assist in clinical decision-making, and improve overall healthcare delivery.
Familiarity with AI in Healthcare
Between July 1 and September 30, 2023, we asked people in our national Market Insights study to report the extent of their familiarity with AI, focusing our analysis on the 38,331 who had a care experience within a year of their response. Only about 1 in 4 say they have a sense of how AI is used in healthcare. Given the rapid pace of AI development, it is probably not safe to assume that the proportion is much higher for people working in healthcare.
A higher proportion of males (32%) than females (23%) say ‘Yes’; the balance flips for ‘Not really’, and the groups are similar for ‘No.’ When it comes to age, about a third of people aged 25-44 and a quarter of those aged 18–24 and 45-54 say ‘Yes’ – the proportion drops precipitously for those aged 55 and older. The ‘Yes’, ‘No’, and ‘Not really’ groups will be referenced throughout the report.
It’s clear that most people are not familiar with AI. At its root, the term AI refers to the use of machines to perform certain tasks that normally require human intelligence to accomplish. But it also helps to go beyond this broad definition and think about the functionalities of AI. Here are three of the most prominent:
- Machine learning – the use of computer programs that learn from data and improve their performance on a task without additional programming
- Image analysis and pattern recognition – the use of computers to process and make sense of visual data such as images or videos
- Natural language processing (NLP) – the use of computers to interpret, comprehend, analyze, and generate human language
AI can facilitate a wide range of tasks.
While articulating the major functionalities of AI is a step toward clarity, it takes tangible examples to illuminate how AI is transforming the experience and delivery of care. Accordingly, we have detailed three use cases, connecting them to the relevant functionalities and summarizing the impact on both patients and healthcare professionals.
When AI applications are integrated into organizational processes (e.g., scheduling, record-keeping, billing) they can increase efficiency, reduce costs, and improve patient experience. While there are many examples of this, AI-based scheduling is a good one, because it can increase access to care while simultaneously optimizing patient flow, increasing clinical efficiency, and reducing wait times.
Care-Team Impact. Demand forecasting based on machine learning can use past appointment data to make predictions about future utilization trends. These forecasts combined with smart scheduling algorithms, can create more optimal appointment timeframes based on a variety of inputs like appointment type, doctor availability, and patient needs/preferences. Also, AI-powered adaptive scheduling systems can adjust schedules and recommend appointment-time changes on the fly when cancellations occur, which helps ensure that timeslots are used efficiently.
Patient Impact. Automated chatbots built using NLP and machine learning – and integrated into the scheduling system itself – create interactive, virtual assistants that help patients schedule, reschedule, or cancel appointments. Chatbots can also provide appointment details like clinic hours or location. Likewise, automated systems can send appointment reminders to reduce the likelihood of no-shows. And machine learning systems can even learn from patients’ behaviors and/or stated preferences (e.g., response to email vs. text) to offer more tailored experiences when it comes to accessing care.
It is increasingly common for doctors to collaborate with AI systems to analyze medical images, resulting in improved diagnostic accuracy. The use of image-recognition algorithms offers an excellent example of how combining the processing power and learning capacity of AI with a physician’s contextual understanding, knowledge of the patient, and creative problem-solving skills can reduce error and improve outcomes.
Care-Team Impact. The number of yearly publications on the topic AI + medical imaging increased by an order of magnitude from 2008-2018. And now giant tech companies like Amazon, NVidia, and Google have thrown their hats in the ring, offering AI-systems built specifically for medical imaging in fields as diverse as dermatology, ophthalmology, and radiology. Google’s DeepMind partnership with Moorfields Eye Hospital in the UK is a well-documented, promising example of what a doctor + AI diagnostic collaboration looks like:
- Training the model. Neural networks were trained to detect eye diseases based on 15,000 eye scans from nearly 7,500 Moorfields patients.
- Testing the model. The model was compared with diagnoses given by 8 doctors. AI was correct 94.5% of the time – equal to some of the retina specialists and better than others.
- New data input. As new scans came in, they were fed through the model, which created outputs on eye-disease detection, severity, and progression.
- Image analysis. Doctors examined outputs and looked for concerning cases as well as faulty analyses.
- Alerts and triage. Rather than deploying AI as a standalone diagnostic tool, the system was used in conjunction with human experts to create alerts and triage cases.
- Collaborative diagnosis. The AI outputs and doctors’ clinical expertise were used to finalize diagnoses and determine the appropriate treatment plan.
Patient Impact. Unaided by AI, image analysis can be laborious, time-consuming, error-prone work; a single hospital can produce hundreds, sometimes thousands of medical imaging scans in a single day. A machine’s ability to crunch through images is astounding in and of itself, but AI models don’t have shift changes, require sleep, or get distracted. Given AI-systems that can accurately accomplish diagnostic tasks that typically occupy a significant amount of a doctor’s time, AI + doctor collaboration potentially allows providers to engage in other important work such as explaining diagnoses, discussing treatment plans, and personalizing care.
Augmented reality (AR) powered by AI is at the cutting edge of innovations in patient care. Multiple AR + AI projects are underway that utilize AR systems for applications as diverse as surgical procedures, medical training and education, and creating interactive visualizations that help patients better understand their conditions and treatment options. In this section we focus on Microsoft’s HoloLens 2 and how it is fundamentally changing the way we deliver personalized medicine.
Care-Team Impact. Generally speaking, Microsoft’s HoloLens 2 is simply an AR system that offers a mixed-reality experience in a variety of arenas. But combined with AI and applied to healthcare, HoloLens 2 is revolutionizing care. For example, neurosurgeons at Providence Swedish in Seattle use HoloLens 2 powered by an AI-driven guidance system called Medivis to prepare for surgery cases. These technologies allow surgeons to develop an individualized operating plan based on each patient’s idiosyncratic anatomy and pathology. It works by taking patient-specific image data from X-rays, PET scans, etc., and superimposing that information onto the patient’s body, in turn creating a visual information system that allows real-time guidance before and during complex procedures – reducing errors and the likelihood that complications will arise during surgery.
Patient Impact. For patients, the benefits of AI-enabled AR systems are not confined to error reduction. For example, research has shown that treatment explanations using mixed-reality tools can significantly increase patient understanding and reduce anxiety compared to analog models. These findings suggest that AR + AI technologies can be used effectively to enhance patient education by providing interactive explanations of their conditions, helping to make difficult medical procedures easier to understand, and enabling more collaborative decision-making/treatment plans.
Familiarity matters.
Current applications and breakthroughs are exciting, but comfort with AI is a direct function of familiarity. Indeed, people who report having a sense of how AI is used in healthcare are markedly more likely to deem it ‘important’ or ‘the next big thing’ as compared to people less familiar with AI who, if they have an opinion, tend to see AI as ‘dangerous.’
Is AI the Next Big Thing?
While all groups are cautious about AI, caution is the watchword for those who are less familiar with it; they also tend to report being frightened by AI in healthcare.
All groups are most sanguine about the relatively ‘simple’ applications of AI in scheduling and answering questions, and these are two very important use cases. While those most familiar with AI are more favorable toward other applications than are their counterparts, there is clearly a lot of work to be done when it comes to helping patients understand the promise of AI across the board.
People trust doctors. They’re less sure about doctors using AI.
In the context of accomplishing clinical tasks, trust in doctors using AI and in AI alone is higher for people who are familiar with AI. That said, it’s striking that even people who profess familiarity with AI place more trust in doctors alone than in doctors using AI – and this pattern becomes more pronounced as people consider the use of AI for diagnosis and treatment, not just interpreting images. Again, there is a major opportunity to align perception with reality, as studies have documented the benefits of AI across a range of clinical and non-clinical healthcare tasks.
Potential Pitfalls
A large proportion of the people in our study expressed caution about AI, regardless of their familiarity with it. Healthy skepticism is certainly warranted, as there are plenty of potential pitfalls where AI in healthcare is concerned. Here are 3 important concerns:
Transparency. AI systems work by crunching vast amounts of data. These data often include medical records, protected health information (PHI), diagnostic images and the like. For example, training image-recognition models typically requires thousands, if not tens of thousands of images or more, depending on the complexity of the imagery. It can also be time-consuming and expensive to generate large image-based datasets, so maintaining (and even sharing) extant datasets is often necessary. This presents myriad challenges for ensuring the deidentification and long-term secure storage of sensitive health information. Further complicating matters, patients may not be aware of – or explicitly consent to – how their data might be used for developing AI applications. As AI becomes more prevalent, building trust by explicitly communicating a commitment to ethical AI practices will become increasingly important. This can only be accomplished through transparency and must include conversations about why and how a patient’s data might be used in an organization’s efforts to utilize AI.
Bias. Another area of deep concern for the ethical use of AI is conscious and unconscious bias in our data, algorithms, outputs, and interpretations. It doesn’t take much effort to find dozens of cautionary tales, wherein well-meaning researchers built an AI model on top of a deeply biased dataset, only to have those biases amplified by their AI outputs. A 2019 paper published in Science found that commercial algorithms widely used by US hospitals to guide care for millions of patients were significantly biased against Black patients because developers used cost as a proxy for need in their model. The bias arose because there is unequal access to care among demographic groups – meaning we spend relatively less money caring for Black patients. The effect was that Black patients were considerably sicker than white patients at a given risk score assigned by the model, which resulted in Black patients being considered healthier than they were, leading to a reduction in much-needed care.
Chatbot Hallucinations. Generative AI tools like ChatGPT are trained to predict strings of words based on massive datasets. However, they lack reasoning and aren’t sensitive to factual inconsistencies or misleading statements. Futurist Bernard Marr describes hallucination in AI as “the generation of outputs that may sound plausible but are either factually incorrect or unrelated to the given context. These outputs often emerge from the AI model’s inherent biases, lack of real-world understanding, or training data limitations.” This is a real issue, given the high stakes, risk of misinformation, and low tolerance for error in healthcare where in fact, people may expect zero error from AI.
Bottom Line
The use of AI in healthcare is in its infancy – ChatGPT was launched a year ago – and while there is certainly reason for optimism around the new technology, we must be transparent about its uses and circumspect about its capabilities. AI can improve care, but it cannot actually care for people. We need to transcend the argument about whether AI will replace health professionals – it won’t. But AI can handle many tasks that will help clinicians be more effective and efficient giving them more time to humanize care for every patient. Indeed, we have agency to decide where, how, and to what extent AI can smooth organizational processes, improve access to care, accelerate accurate diagnoses, and deliver more personalized treatment plans. Some health organizations will be farther ahead than others, but all would do well to start educating their internal and external audiences about AI, and their vision for using it. Now.
Explore additional nSight reports to get insider data and perspectives you need to drive strategic change. Discover More.
Explore additional nSight reports to get insider data and perspectives you need to drive strategic change. Discover More.
© NRC Health 2023
Suggested citation for this report:
England W, Makoul G. 2023. AI in Healthcare: Promise and Pitfalls. Human Understanding Institute – NRC Health. https://go.nrchealth.com/ai-in-healthcare-promise-and-pitfalls-citation (Accessed mm/dd/yyyy).
© NRC Health 2023